The transition to a circular economy, the re-use of products, requires an efficient collection and identification of used products. What do they consist of? What is usable? Every product is unique due to its history. Nevertheless, it is often similar to its successors. To facilitate identification, an artificial intelligence (AI) supports the identification of the product in the "EIBA" project.
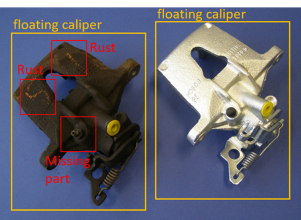
Teamwork with artificial intelligence
At the end of a utilization phase, there are various disposal or processing strategies for a product. Depending on their type and condition, products can be recycled or processed and reused. For this purpose, they must be clearly identified and evaluated. The challenge here is that many product models differ only slightly from one another and are difficult to identify due to contamination and wear. In addition, the professional personnel only has a few seconds to identify and evaluate them.
In order to support people in their work or to train new employees, EIBA’s project consortium wants to provide them with a machine. It is supposed to support looking at and evaluating the product. Sensor-based data is evaluated with the help of artificial intelligence in combination with other information and formulated into a decision recommendation. Thanks to the dual control principle of man and machine, both the error rare during identification and the strain on people is to be reduced.
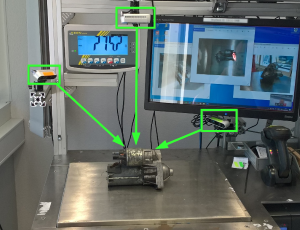
Self-learning technology
The aim of the “EIBA” project is to develop a system for the identification and condition assessment of used parts. This will make an important contribution to closing the cycle through digital technologies. By using methods of artificial intelligence – such as machine learning as well as deep learning – the system should be able to identify products and compare them with other available information. By continuously expanding the data, it should also be able to adapt to new products and requirements.
One innovation of the project is to complementary combine the competences of man and machine to enable higher process security and efficiency. The resulting system will be analysed according to sustainability aspects: What has changed for the worker? What additional environmental burdens are initially caused by the use of machines, and how great are the environmental benefits gained through increased efficiency?
Results (Status June 2022)
The development of the system is based on the example of used car parts (cores). In order to include also challenges and requirements of other products in the development-process, extensive interviews with companies of other industries such as textiles, printer cartridges or semiconductors were conducted. An important focus in the project is machine vision. For a proof-of-concept in that field, based on image data of approx. 1400 different used parts, the AI was able to identify more than 98% correctly in performance tests. As these performance tests have been conducted in controlled conditions, validation in industrial environment is pending. To enable that all workstations in a C-ECO location have been equipped with cameras and digital scales and have been connected to the identification-software. With this setting, data availability of RGB- and depth-images as well as weight-data will be continuously enhanced. This generates further training data to improve the AI’s capabilities and to adapt it better to „real life” conditions. At the moment, 3,500 used car parts with 21,000 data sets (124,000 images) have already been digitized in the project.
In addition to image recognition, statistical evaluation methods were implemented and tested to generate identification suggestions for the workers from historical delivery data and weight-data and package information. The performance tests of the statistical models identified 66% of the parts correctly using 213,879 real measurements. An additional challenge is the efficient integration of the sensors and AI in the working process. To do so the current process has been analyzed in detail to create a concept for the adaption. Here the focus is on the presentation of the AI results in the human-machine-interface and on restructuring the process steps using the new available data. These concepts were implemented in clickable digital demonstrators and prepared for implementation in productive environment at C-ECO. The interpreted digital sensor data, statistical evaluation and the human operators input are now merged for the optimal support of used parts identification making best use of the specific capabilities of each approach.
Based on the recognition rates, the effects on CO2 emissions of the sorting were calculated, using the example of starters. This showed that the savings due to additional parts going to remanufacturing are significantly higher than the additional emissions caused by the use of the AI. The additional energy expenditure resulting from the use of the AI and the additional sensors was investigated and put in relation to the improved sorting rate due to the use of AI. Looking only on starter, the results show that the additional energy expenditure for training and use of the AI pays off after just one day of sorting due to the additional parts that are available for remanufacturing. In total, there was a potential of saving CO2-equivalent emissions of 27.5 tons solely considering the improved sorting of starter motors.
Interdisciplinary teamwork
The challenges in the “EIBA” project are a result of the complex combination of the latest information
technology, application-oriented process know-how and knowledge of market requirements for an efficient circular economy which can be globally scaled. To address that, engineers from different disciplines work together to look at the challenges from different perspectives and make the best possible use of the potentials.
Publications
Final Report (short, German, 2023)
Project flyer of the funding measure (German / English) (March 2021)
The project flyers offer an insight into the contents and goals of the ReziProK projects and present first results in each case.
Project sheets of the funding measure (German) (September 2019)
The project sheets provide a brief overview of the individual projects and their goals.
Contributions to the ReziProK Transfer Conference in June 2022
Poster (German) (June 2022)
Presentation (German) (June 2022)
Contributions to the ReziProK Kick-off event in December 2019
Poster - in German (December 2019)
Presentation - in German (December 2019)
Picture credits: BOSCH/C-ECO